5 Major Applications of Predictive Analytics in Retail
Retail is a data-rich industry. Retailers interact with millions of customers every year and produce huge volumes of data. Catering to the needs of these customers while being proactive in the highly competitive market is challenging. However, retailers can use data in their possession to their advantage by leveraging predictive analytics. Let us explore five major applications of predictive analytics in Retail.
In our previous blogs, we have covered how predictive analytics work for Manufacturing, Healthcare, Finance, and Insurance.
Applications of predictive analytics in Retail
Delivering personalization to customers
If you look at reviews for a retailer on Google, you may find negative reviews and 1-star ratings for inconveniences and unpleasant experiences customers might have faced. As a shopper, you often count these reviews and ratings in your purchase decisions, don’t you?
Customer experience is key. It directly impacts brand loyalty and your business success. As far as CX is concerned, personalization is the holy grail. A McKinsey survey found that 80% of adults want personalization from retailers. Once only limited to offers, personalization has now extended to every touchpoint. When they receive personalized messages, recommendations, and offers, your customers feel valued and become loyal customers. McKinsey’s survey also found that when done right, personalization can lift sales and reduce marketing costs by 10-20%.

The Next Level of Personalization in Retail (bcg.com)
For most retailers, the question remains – how to deliver personalization?
As a retailer, you possess an abundance of customer and business data. You can process this data using artificial intelligence and machine learning technologies to gain deeper insights into your customer preferences, needs, and shopping behaviors. You can use these insights to make tailored recommendations that your customers are more likely to buy.
For example, Target ran a campaign where they leveraged customer shopping data. Based on customers’ shopping history, they identified parents-to-be and pushed tailored recommendations to them. Based on the type and quantity of items bought, Target could predict due dates and push tailored offers on baby products. This predictive analytics-based strategy helped the company increase sales and grow revenue by over 50%.
Implementing optimal pricing strategy
Pricing plays an important role in influencing purchase decisions. Lower prices attract sales but bring your revenues down. Higher prices can turn your customers away. You need to strike a balance between profitability and competition and set a price that your customers are willing to pay. For most retailers, this is a challenge. They grapple with setting optimal prices while accounting for demand fluctuations and changing market dynamics.
When setting a price, you need to consider the demand for your product, the pricing strategy of your competitors, the target demography, and the behavior of the customers. Using predictive analytics, you can analyze demand patterns and competitors’ pricing strategies. So you can understand market dynamics better and accurately forecast demand.
Predictive analytics will help you understand the price sensitivity of your customers and segment them effectively. So, you don’t need to follow the same size fits all approach. Predictive analytics can help you bring personalization to pricing. You can customize pricing for each segment of customers and boost sales while maximizing profitability.
Maintaining optimized inventory levels
For retailers, both overstocking and understocking are a nightmare. Overstocking eats into your profits. As per TotalRetail Studies found that retailers lost $300 billion in revenues due to markdowns resulting from overbuying. On the other hand, understocking turns your customers away and results in lost revenue. The out-of-stock boards cost retailers $82 billion in 2021.
Predictive analytics revolutionizes inventory management in retail by providing actionable insights to tackle complexities. Retailers often grapple with overstocking or stockouts due to uncertain demand and supply variations. Predictive analytics leverages historical sales data and seasonal trends to forecast demand accurately. This enables retailers to maintain optimal stock levels, preventing excess inventory costs and lost sales opportunities.
By anticipating demand fluctuations and trends, retailers can align procurement and distribution more efficiently. This will eliminate operational inefficiencies. Predictive analytics transforms inventory management from reactive to proactive. You can ensure products are available when and where customers need them and enhance customer satisfaction.
Revenue forecasting for better decision making
Revenue forecasting in Retail involves several factors, including changing consumer behavior, demand fluctuations, and economic shift. The complexities in predicting these factors create a challenge for retailers in forecasting revenue.
Predictive analytics offers a solution by leveraging historical sales data and customer behavior insights. By employing advanced algorithms and machine learning techniques, retailers can better understand consumer preferences and buying patterns. These models can identify hidden correlations between various factors, such as weather, promotions, and social trends, allowing for more precise predictions.
Using predictive analytics, retailers can perform scenario analysis, assessing the potential impact of different strategies on revenue. Retailers can adjust their forecasts based on real-time data and adjust their operations accordingly. This adaptive approach ensures agility in responding to market changes and optimizing revenue opportunities.
Fighting against evolving fraud techniques
Fraud in Retail includes activities such as payment fraud, account takeovers, identity theft, and returns fraud. These fraudulent actions can lead to financial losses and damage brand reputation. National Retail Federation reported that US retailers lost $7.8 billion due to fraudulent returns.
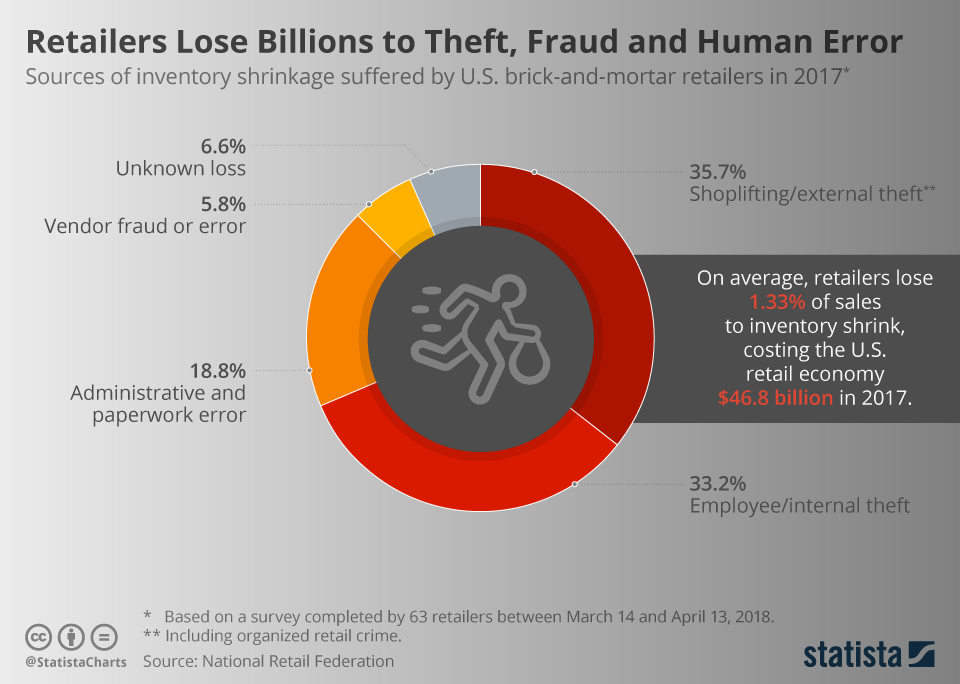
(reference: Chart: Retailers Lose Billions to Theft, Fraud, and Human Error | Statista)
Fraud tactics are evolving over time. Conventional rule-based fraud detection systems often struggle to keep up with these trends. This is where predictive analytics comes into play.
Predictive analytics assists retailers in combating fraud by analyzing large volumes of transactional and behavioral data to identify unusual patterns and anomalies. By establishing baseline behavior for legitimate transactions, predictive models can swiftly detect deviations that might indicate fraudulent activities.
Predictive analytics use machine learning algorithms, which continuously learn from historical data and identify emerging fraud patterns. This adaptive approach enables retailers to stay ahead of fraudsters who constantly modify their tactics.
Predictive analytics also helps retailers reduce false positives, ensuring that legitimate transactions are not mistakenly flagged as fraudulent. By using a more nuanced and data-driven approach, retailers can maintain a seamless customer experience while still identifying potential threats. Retailers can track and detect fraudulent actions in real-time by analyzing transactions as they happen. This enables them to flag or block suspicious activities and minimize potential losses.
Want to leverage predictive analytics in retail?
As a retailer, you collect data from different sources in different formats. Integrating this data, cleansing and processing it, and extracting predictive analytics can be overwhelming for you. At Saxon AI, we do the hard work for you. We offer data, and analytics managed services that will empower you with predictive analytics, easily accessible on real-time dashboards.
Want to get started quickly? Explore InsightBox – our data and analytics accelerator.
Want to talk to our data experts? Contact us.
You can follow us on LinkedIn and Medium to never miss an update.
Comments
Post a Comment